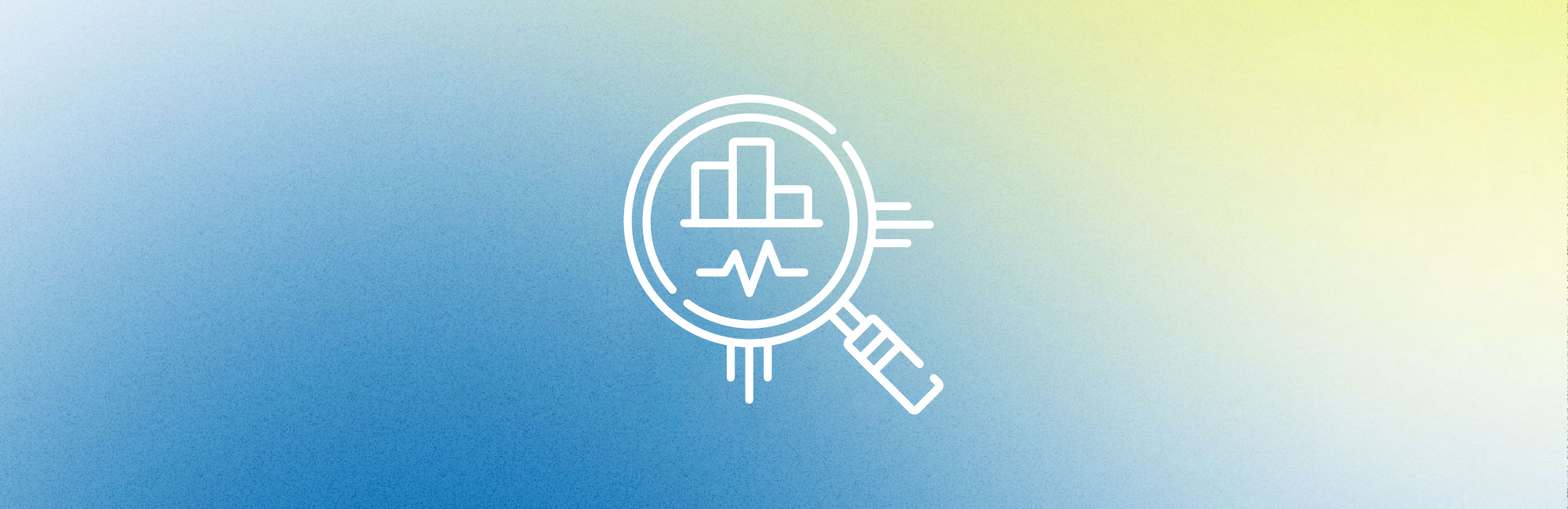
Maintenance has always played a crucial role in ensuring the reliability and efficiency of equipment and machinery. However traditional maintenance strategies, such as routine maintenance or reactive maintenance can be costly and inefficient. Thankfully, with the growing use of data analytics, predictive maintenance has emerged as a game-changer. By harnessing the power of data, organizations are optimizing their predictive maintenance schedules, reducing downtime, and cutting operational costs. As technology continues to advance, data-driven predictive maintenance is poised to revolutionize equipment management, making it an indispensable tool for organizations across industries.
Leveraging data-driven predictive maintenance
Predictive maintenance is a proactive maintenance strategy that relies on data and analytics to determine when equipment or machinery is likely to fail. By collecting and analyzing data from various sensors, IoT devices, and other sources, it allows organizations to anticipate maintenance needs and schedule them at the right time. This can:
Reduce downtime: By addressing maintenance issues before they become critical, businesses can significantly reduce unplanned downtime, improving overall operational efficiency.
Increase cost savings: Efficient use of maintenance resources and reduced emergency repairs result in cost savings.
Prolong asset lifespan: Regular, timely maintenance extends the lifespan of equipment, reducing the need for frequent replacements.
Enhance safety: Predictive maintenance can help prevent accidents and hazards associated with equipment failures.
Improve data-driven decision-making: With data analytics, businesses gain valuable insights into their operations, enabling better decision-making and long-term planning.
Building a data-powered predictive maintenance program: Step-by-step guide
Whether you are an experienced professional or just beginning your maintenance optimization journey, having a well-planned roadmap can help you understand the key components of developing a data-driven predictive maintenance program. To help you get started, here is a step-by-step guide to implementing a successful data-powered predictive maintenance program:
Data collection and integration
The foundation of predictive maintenance lies in collecting relevant data. Sensors and IoT devices are typically used to monitor equipment performance, temperature, vibration, and other critical parameters. The first step is therefore to ensure that all necessary data is collected and integrated into a centralized system. This may involve setting up IoT infrastructure and implementing data integration tools.
Data preprocessing and cleaning
Raw data often contains noise and inconsistencies. To make meaningful predictions, data preprocessing and cleaning are essential. This should include data normalization, removing outliers, and handling missing data. The aim should be to transform data into a format suitable for analysis.
Data analysis and machine learning
With clean and normalized data in hand, data analysis techniques and machine learning algorithms can be applied. Algorithms such as regression, decision trees, and neural networks can help identify patterns and correlations in the data. These patterns are used to predict when maintenance is required.
Anomaly detection
Anomaly detection algorithms are crucial in identifying deviations from normal equipment behavior. By setting thresholds and rules, the system can detect anomalies that may indicate impending failures. This allows for timely intervention.
Predictive models development
The heart of predictive maintenance is the creation of predictive models. These models use historical data to predict future equipment behavior. Models can be fine-tuned and trained over time, becoming more accurate in predicting maintenance needs.
Scheduling optimization
Once the predictive models are in place, organizations can optimize maintenance schedules. They can prioritize maintenance based on the severity of predictions and the impact on operations. This ensures that maintenance resources are allocated efficiently.
Continuous improvement
Predictive maintenance is an iterative process. It is essential to continually monitor the system's performance and fine-tune the models as needed. As new data becomes available, the system should adapt and improve its predictions.
TMA Systems: Your trusted partner for data-enabled predictive maintenance
With a proven track record of delivering innovative solutions, TMA Systems harnesses the power of data analytics to transform traditional maintenance practices into proactive, efficient, and cost-effective processes. By leveraging predictive maintenance techniques, we help businesses anticipate and address equipment issues before they become critical, reducing downtime and saving valuable resources. Our commitment to excellence, powered by WebTMA — our maintenance management solution can optimize maintenance operations and extend the lifespan of assets. Embrace the future of maintenance with TMA Systems and unlock a world of possibilities for optimizing your facility and asset management. Contact us now to know more.
Ready for a Demo?
Interested in taking this to the next level? Sign up for a free demo with a TMA Systems Account Executive. Enter your email below and we will reserve a time for you to test drive WebTMA, or call us at 1.800.862.1130!
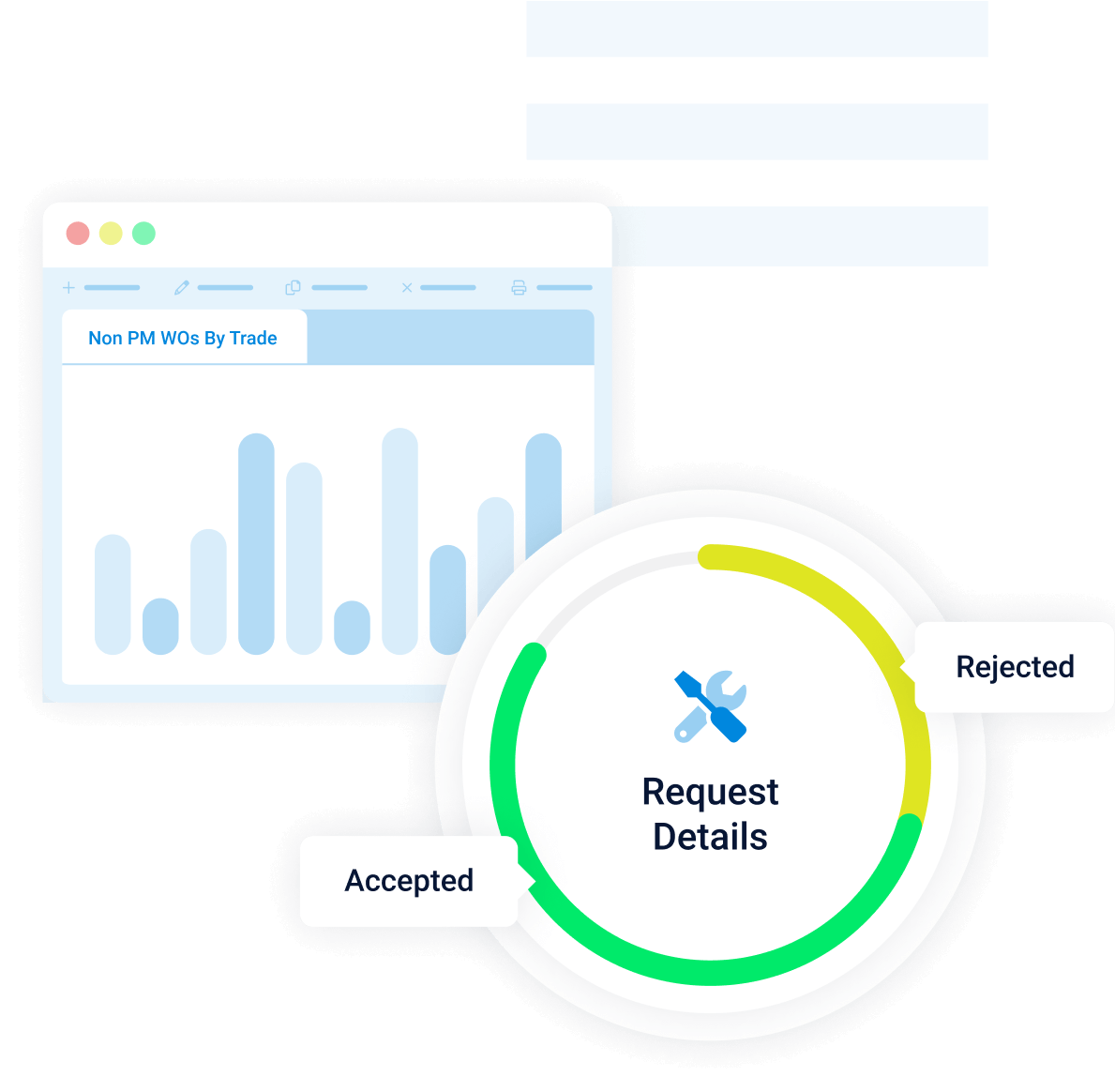

